In the first quarter of 2020, the world-leading stock indexes crashed, sometimes losing over 12% in a single day, due to the global pandemic. In the same period, Netflix’s stocks grew by almost 14%. As global lockdowns keep people home, it’s no surprise that Netflix’s viewership skyrocketed. The question is: how has Netflix positioned itself as the go-to place for streaming videos? As I outline in my latest book, this is largely thanks to their mastery of data analytics and learning systems.
The increasing digitization of business models is leading our systems of production into a Fourth Industrial Revolution, an age of rapid change in the way companies succeed. To help companies navigate this new industrial revolution, I’ve embarked on a three-year long research project of over seventy case studies worldwide. The result is a framework called C.L.E.V.E.R., which is the acronym for six drivers that determine strategic success during this new industrial revolution.
These strategic drivers are: Collaborative Intelligence, Learning Systems, Exponential Technologies, Value Facilitation, Ethical Championship and Responsive Decision-Making.
“The C.L.E.V.E.R. framework by Alessandro Lanteri, opened our eyes by giving us a clear view of the drivers that could shape our future.”
Giuseppe Giachetti, CEO of Ludocards
What’s Netflix doing?
Netflix tracks the content its viewers watch and like, the movies they stop watching, the moments when they fast-forward or rewind—this is all data. Netflix gathers and analyzes it to extract information about the best content for you. With such information, Netflix’s algorithms make automatic decisions such as recommending other content that you are likely to watch. They have become so accurate that 80% of the content streamed on the platform comes from recommendations.
Most people refer to algorithms that perform automatic actions as Artificial Intelligence (AI). AI are algorithms that process data to generate outputs that appear to be intelligent by human standards. For example, they can quickly calculate the fastest route from A to B, accounting for realtime traffic information. It would be an impressive feat of intelligence for a human to do the same. Yet, the very same algorithms can sometimes fail drivers, leading them into a muddy field. As said, algorithms usually appear to be intelligent, but they aren’t really. This is why I don’t like to talk about AI, and I prefer Learning Systems.
What are learning systems?
Algorithms can autonomously identify the features of certain objects and recognize which features these objects have in common. For example, image recognition algorithms learn to associate your photos with your name. Whenever you upload a new photo the algorithms identify the features and automatically associate it with your name. Each time algorithms analyze a new photo they get better. That’s learning.
How do these systems learn?
The main approaches to learning systems are: supervised learning, unsupervised learning, and reinforcement learning.
- Supervised learning involves training algorithms with many examples of an object (e.g. photos, sounds, words) accompanied by a human-generated tag (e.g. a caption, a music genre, a translation into Italian). For example, after being trained to identify the features of emails users tagged as spam, Gmail recognizes undesired emails and automatically sends them to the junk folder.
- In unsupervised learning, no tag is provided. Algorithms parse the data and identify patterns humans cannot spot. For example, Ant Financial identified a user group that spent more money than others fixing their mobile screens. These same users also spent more money than others on tight jeans. A human could not see this correlation. Ant Financial now offers a new screen insurance targeted at females who wear tight jeans.
- A variant of unsupervised learning is reinforcement learning. After specifying the rules of the game and the standards of successful performance, algorithms learn how to succeed by trial and error. This is how AlphaGo defeated the human grandmaster Lee Seedol at the ancient game of Go.
As we increasingly rely on algorithms that learn on their own, one of the techniques used to improve their performance is deep learning. Deep learning algorithms analyze features of datasets one at a time, developing deeper layers of analysis, hence the name. This technique can be applied to each of the three learning styles above.
Figure source: A. Lanteri (2019), CLEVER. The Six Strategic Drivers for the Fourth Industrial Revolution. Lioncrest.
Data is the new oil
Learning systems need examples to learn, their performance depends on the data available. Data is becoming more accessible, thanks to our increased ability to capture, store, and analyze it in real-time. With the right data, learning systems can transform information companies have into information they don’t have. So, data has become a new type of fuel that powers automatic decisions critical to digital business models like that of Netflix.
How many versions of Netflix do you think exist?
Netflix uses Learning Systems to great effect. By aggregating data from its 167 million subscribers, Netflix plans and produces new content its viewers want to watch. The world-famous series “House of Cards” was developed on the basis of viewers’ ratings. Unlike its competitors, Netflix didn’t need a pilot episode for the series, because the theme, the lead actor, and the director had already been validated by millions of users. The success rates of traditional TV shows are 30%-40%. Netflix original shows’ are 80%.
Netflix understands its viewers so well, that each enjoys a uniquely customized experience. In 2013, it stated that “there are thirty-three million different versions of Netflix.” At the time, there were thirty-three million subscribers.
You could rightly believe that today Netflix offers 167 million different customized versions. That would overlook the observation that data shows “different viewing behavior depending on the day of the week, the time of the day, the device, and sometimes even the location.” Moreover, Netflix constantly tests different recommendations to different users, sometimes even developing different trailers for different users, in order to gauge what content works best. This means that Netflix now has more versions than it has subscribers.
Embracing learning systems prepared Netflix for the Fourth Industrial Revolution and keeps it thriving through troubled times.
Learn to thrive in times of constant change with a pioneering MBA or business masters from Hult International Business School.
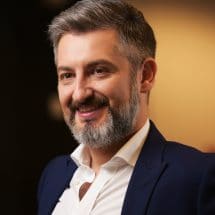
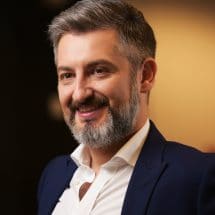